- Full Stack Express
- Posts
- The Creation of Tinder’s Design System Obsidian
The Creation of Tinder’s Design System Obsidian

🌙 Hello world ☀️
Exciting News! TanStack Query v5.0.0 has officially launched after an intense year of development, improving from v4 with a streamlined API and size reduction of around 20%. New features include simplified optimistic updates, 1st class suspense support, sharable mutation state, and enhanced Devtools.
In this week’s email:
Design System: Learn how Tinder created Obsidian.
Machine Learning: Understand how Expedia uses price alerts to increase engagement with their users.
React: Million.js compiles React components, reducing virtual DOM use and cutting memory usage by 55%.
Node.js: Improve Node.js application performance by leveraging the cluster module for multi-instance concurrency and scaling.
Next.js: Optimized barrel file imports resulted in 70% faster development, 28% quicker builds, and 40% improved cold starts.
Productivity: Managing capacity instead of time to do our best work.
If debugging is the process of removing software bugs, then programming must be the process of putting them in.
DESIGN SYSTEM
The Creation of Tinder’s Design System Obsidian
Introduction
In 2012, Tinder introduced an innovative approach to online dating with its Swipe Right® and Swipe Left™ features. This intuitive design quickly gained traction, leading to the app's immense popularity.
As Tinder grew over the decade, the design complexities expanded, and a design overhaul became essential for coherence and consistency.
The Challenge
Tinder's initial success led to swift feature introductions. This rapid expansion, while essential for keeping user engagement high, resulted in a design language that began to diverge in different parts of the app.
Not only did this create challenges in maintaining a unified user experience, but it also led to increased costs in design adaptations and a more intricate codebase.

Difficulty Maintaining Styles
The Obsidian Solution
In response to the growing design challenges, Tinder conceptualized 'Obsidian'. This new system was intended to be a design ecosystem composed of standardized design tokens, reusable UI components, documentation, and design tools.
Design Tokens: These became the building blocks of Obsidian. Design Tokens were standardized references for every design aspect ranging from colors to fonts.
Tokens Studio, A Figma Plugin
Base Tokens: These were direct design references, pointing to specifics such as exact color shades or font measurements.
Context Tokens: To provide adaptability, especially in scenarios like shifting between dark and light modes, context tokens were introduced.
Compatibility with Prevailing Tools: The Obsidian system was not built in isolation. It effectively integrated existing tools like Tokens Studio on Figma for defining design tokens. Moreover, to ensure consistent representation of design aspects across various platforms like iOS, Android, and the Web, Style Dictionary was employed.
To support designers and developers in adapting to Obsidian, Tinder went the extra mile:
A detailed guide was crafted using Zeroheight to help teams navigate the nuances of the new system.
Design Documentation Created Using Zeroheight
A token lookup tool was introduced. This handy tool streamlined the process of selecting and employing design elements.
Tinder’s Token Lookup Tool
Impact & Results
The benefits of Obsidian were immediately noticeable:
Uniform Design: Features such as the sexual orientation selection screen, once fragmented in design, now showcased greater uniformity and accessibility.
Feedback from the Trenches: Senior iOS engineer at Tinder, Siddharthan Asokan, was particularly impressed. He emphasized the reduced time spent in design deliberations and how Obsidian made design implementation remarkably straightforward.
P.S. If you’re enjoying the content of this newsletter, please share it with your network: https://www.fullstackexpress.io/subscribe
MACHINE LEARNING
How Expedia Uses Price Alerts to Increase Engagement
Expedia Group (EG) offers travelers optimal deals using technology like flight price alerts. These alerts notify users of price changes, allowing bookings at desired prices.

Expedia Price Alerts
Expedia’s transition from business rule-driven alerts to machine learning (ML) has helped improve traveler engagement by delivering more precise notifications and avoiding over-communication.
Message Relevancy (MR) Model
Price alerts, originally based on rigid business rules, lacked flexibility. In contrast, the ML-driven approach tailors notifications for a more enhanced user experience.
By analyzing data on subscriptions, flight routes, and user behavior, the system anticipates user engagement, emphasizing metrics like recall and alert open rates.
Key aspects incorporated:
Outcome Objective: Ensure alerts meet traveler's financial goals while also optimizing business costs.
Data Usage: Data about subscriptions, interactions, routes, and flight price changes are used to predict if a traveler will open a notification.
Success Metrics: Focused on recall metric and business metrics like subscriber retention and notification open rates.
Techniques and Optimizations: Used Random Forest Classifier for its simplicity and later introduced improvements like automated model tuning to increase efficiency.
Model Architecture and Improvements
The model was built on the Random Forest Classifier and later expanded to include different types of "opens" as targets.

General Random Forest Classifier Architecture
Enhancements:
Automated Model Tuning (AMT) was used for better hyperparameter tuning, leading to a 20% improvement in the F1 score.
Moved from scikit-learn's RandomizedSearchCV to AMT for better optimization and efficient model training.
Safeguard Rules:
Implemented to ensure a wider subscriber base receives notifications, such as sending alerts to long-time subscribers or when price changes cross a certain threshold.
The improved model provides timely notifications from the second day of a traveler's subscription.
Results
The introduction of the MR model brought notable improvements:
Increase in the opt-in rate for price alerts.
More subscribers are receiving and opening notifications.
Demonstrates the model's positive impact on the price alerts campaign, ensuring better engagement and satisfaction among travelers.
DATA STRUCTURES & ALGORITHMS
Best Time to Buy and Sell Stock
Missed the solutions to this week’s coding workout?
Learn how to solve this common interview question here.
Million.js is a tool designed to optimize React applications, making them more memory-efficient.
Traditional React apps use the virtual DOM to represent UI components, which can lead to large nested JavaScript objects that consume significant memory, especially in complex component trees.This becomes a challenge with continuous object diffing, garbage collection, and potential memory leaks.
Million.js addresses these issues by compiling React components into optimized Higher Order Components, reducing the reliance on the virtual DOM, and leading to a reduction in memory usage by approximately 55% compared to standard React, offering performance closer to vanilla JavaScript.
In Node.js, by default, applications run on a single CPU thread, which can lead to performance issues for CPU-intensive tasks.
To address this, Node.js offers the cluster module, allowing for the creation of multiple application instances running concurrently on a machine. This module also features a built-in load balancer using the round-robin algorithm to distribute incoming requests among the instances.
This tutorial teaches how to implement clustering in a Node.js app, enhance scalability with the pm2 module, and evaluate performance differences using load testing tools.
In the recent version of Next.js, significant optimizations were made to package imports, leading to quicker local development and improved production cold starts.
The optimization specifically addresses the inefficiencies of barrel files in JavaScript, which are used for grouping and exporting multiple modules. Traditional methods, like tree-shaking, have limitations, so Next.js introduced the "optimizePackageImports" option to auto-analyze and map imports.
This change has resulted in up to 70% faster development times, 28% faster production builds, and 40% quicker cold starts.
PRODUCTIVITY
Manage Your Capacity, Not Your Time
Managing your capacity, which is influenced by energy levels, is more crucial than just managing time. Being mindful of tasks that drain or boost your energy can optimize productivity and prevent burnout. To enhance efficiency, periodically reflect on your work patterns and balance deep work with necessary breaks.
JAVASCRIPT ECOSYSTEM
JS Weekly Pulse
📢 Node.js 21 is now available. The release features an updated V8 engine, introduces stable WebStreams, offers a flag to switch module defaults, and enhances the test runner for functional tests.
📢 API improvements for working with files in the browser.
🚀 SolidJS 1.8.0 - Enhanced streaming serialization, deep data de-duping, improved hydration, reduced template sizes, and improved global script management.
🚀 Electron 27.0.0 - Upgrades to Chromium 118.0.5993.32, Node 18.4.0, and V8 11.8.
🚀 Fresh 1.5 - Partials, improved client-side navigation, UI enhancements.
🚀 Bun 1.0.6 - Addresses regression impacting Docker usage with Bun and implements support for the
overrides
&resolutions
fields inpackage.json
.🚀 TanStack 5.0.0 - Simplified optimistic updates, sharable mutation state, suspense support, and more.
🚀 Parcel 2.10 - Big performance improvements, an improved
--lazy
mode, reduced runtime bundle sizes, and web extension improvements.
RECOMMENDATIONS
To-Do List
✅ Explore: Tesla releases FleetAPI is a RESTful data and command service providing access to Tesla vehicles and energy devices
✅ Learn: Growing as an engineer at Stripe.
✅ Watch: How Spotify manages software at scale. Kelsey Hightower talks with Niklas Gustavsson about Fleet.
✅ Listen: The future of HTTP with Nick Shadrin and Roman Arutyunyan, software architect and principal software engineer at Nginx.
✅ Network: PayPal Developer meetup at Money 20/20 in Las Vegas, October 23rd.
COMMUNITY SPOTLIGHT
Hot Picks in the Dev Community
Viselect - Lightweight library delivering high performance, offering a visual method for element selection similar to desktop interfaces.
OXC - A high-performance toolkit for JavaScript and TypeScript, written in Rust.
Nostalgist - A JavaScript library used for running emulators of retro consoles inside browsers.
Cockpit - Web-based graphical interface for servers.
MEME
If ChatGPT Was A Person…
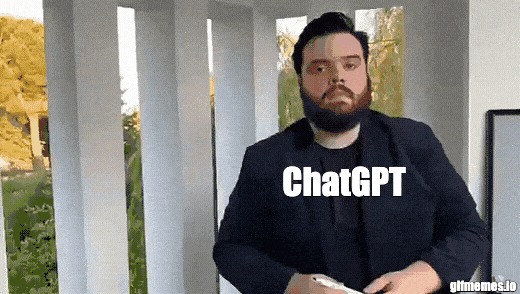
Reply