- Full Stack Express
- Posts
- Why Apple is Quietly Winning the AI Race
Why Apple is Quietly Winning the AI Race


Good morning and welcome back to another edition of Full Stack Express, your weekly newsletter packed with both technical and non-technical tips, the latest tech news, insightful articles, and real-world case studies.
Here’s the notable announcements for the week:
The Node.js team releases version 20.6.0, adding built-in support for
.env
files.The Svelte team releases SvelteKit v1.24, adding support for view transitions (view transitions are in right now).
The open source Git project releases Git 2.42, containing interesting features such as excluding references by pattern.
Astro is launching Astro Studio in 2024, a globally-distributed edge data platform. Join the waitlist here.
We’ll also do deep dives into:
Apple’s AI Breakthrough in Smarter Recommendations
The Intricacies of how Slack Manages Billions of Requests Daily
Why Developers Love Rust: The Rise of Tech’s Most Desired Language
(Bonus) How LinkedIn Automates Application Security for a Safer User Experience
And finish off with:
Byte-sized topics of trending tech news
Interesting tools and packages created by the community
Tip of the week (Kubernetes)
Meme of the week
APPLE’S AI BREAKTHROUGH IN SMARTER RECOMMENDATIONS

Ever wondered how Netflix knows you'll love that new sci-fi series or how Amazon predicts you need a new coffee maker?
The secret sauce is often "Collaborative Filtering," a technique that analyzes your behavior to recommend items you'll likely enjoy.
But Apple is taking this to a whole new level with their groundbreaking model—Sliced Anti-symmetric Decomposition (SAD).

SAD: Sliced Anti-symmetric Decomposition for Collaborative Filtering
Traditional methods use something called "latent vectors" to understand both you and the items you interact with.
But SAD adds a twist—an extra latent vector for each item, making the algorithm smarter and more nuanced.
What makes SAD so special?
Apple's genius lies in a three-dimensional approach to user-item interactions.
Imagine it as a 3D chessboard where every move you make helps the algorithm understand not just what you like, but how you compare multiple items.
This results in more accurate and personalized recommendations.
So why exactly does Apple’s model stand out?
When the additional vector in SAD is set to 1, it's like any other state-of-the-art (SOTA) collaborative filtering model. But here's the kicker: Apple lets this value be driven by actual data.
This opens up a world of possibilities, suggesting that our decision-making might be more complex than previously thought.

SAD vs Traditional Collaborative Filterings
Ever find yourself stuck in a loop, comparing two or three items endlessly? SAD gets that, and it's built to understand these cycles.
The proof is in the numbers.
Apple didn't just make claims; they put SAD to the test.

Test Results for Different Parameters (e.g. Learning Rate)
With over a million user-item interactions from both simulated and real-world datasets, SAD didn't just meet the bar—it raised it.
Outperforming seven SOTA models, SAD proved to be a juggernaut in delivering consistent, highly accurate recommendations.
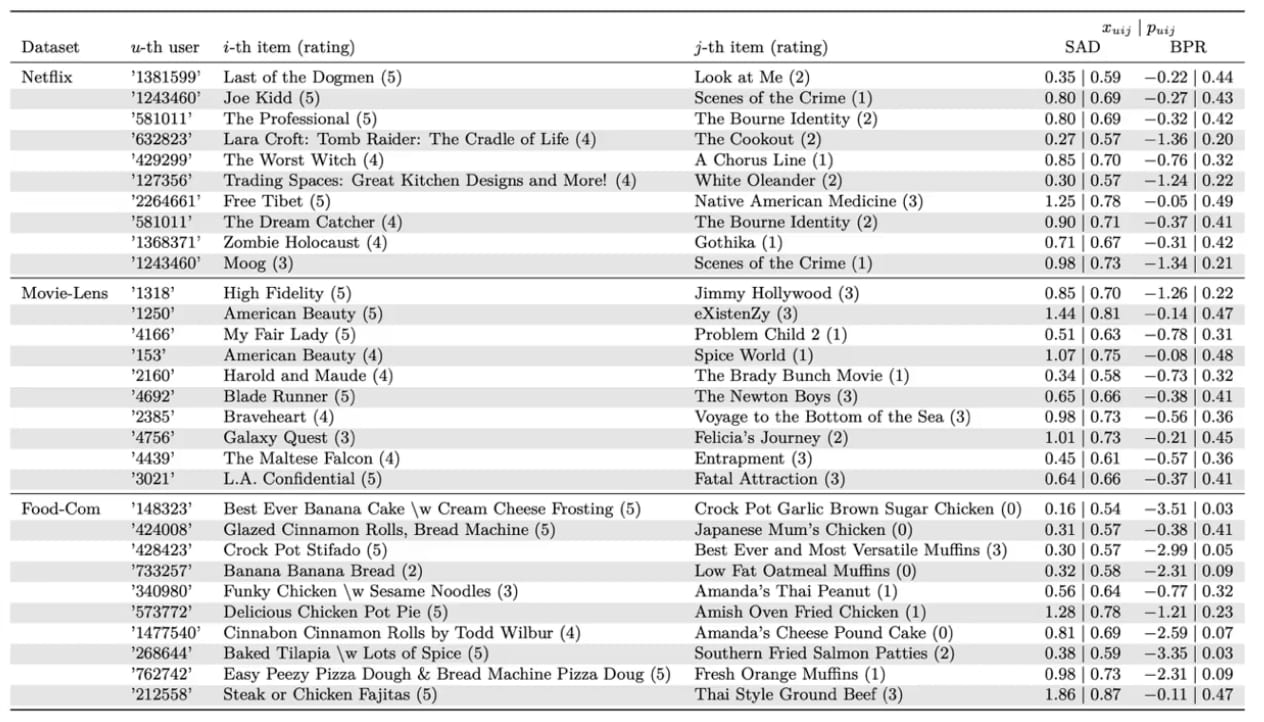
SAD outperforming other models in consistency and accuracy
With a whopping $22.6 billion invested in AI and machine learning, Apple may be quiet, but they're far from inactive.
Between their cutting-edge research and massive R&D investments, it's clear: Apple is gearing up to redefine the future of personalization.
So, the next time your device suggests something eerily perfect, you'll know that Apple's SAD algorithm is the wizard behind the curtain.
Find the full publication here.
Find the model and inference algorithms here.
Find the full in-depth analysis here.
THE INTRICACIES OF HOW SLACK MANAGES BILLIONS OF REQUESTS DAILY

How exactly does Slack juggle billions of network requests daily?
The answer starts with Slack's Edge Points of Presence (PoPs)—a global network of data centers designed to minimize latency and maximize performance.
These PoPs connect to Slack's main AWS region, where core services and storage reside.
The architecture is robust and built for resilience, ensuring a seamless Slack experience even if a data center goes down.

How Requests Flow Through Slack’s Infrastructure
When you interact with Slack, your requests fall into two main categories:
WebSocket Requests: The engine behind real-time messaging
Non-WebSocket Requests: All other functionalities, like file uploads and API calls
Slack uses Amazon Route53 and NS1 to manage domain name resolution, making sure your requests find their way through the network maze efficiently.
WebSocket Traffic for Slack Messages
WebSocket connections are the backbone of Slack's real-time messaging.
Managed by a system called "envoy-wss," these connections ensure that your messages are delivered almost instantly via Slack’s DNS domains.

How Slack Handles WebSocket Traffic
These DNS records are managed by NS1 through a series of filters called Filter Chains.
This sequence directs your messages to the closest Network Load Balancers (NLBs), optimizing for speed and reliability.

Filter Chains
The request is finally directed to either Gatewayserver or Applink based on its parameters.
Gatewayserver is an internal service that maintains WebSocket connections at Slack.
Applink is an internal service designed to lighten the burden of security for customers when building apps that need to receive data.
Slack’s API and Integration Ecosystem
Slack isn't just about messaging. It's also a hub for:
Slack API
Slackbot
Webhooks
Third-party applications
These types of traffic are managed by a dedicated set of servers known as "envoy-edge," fine-tuned for handling heavy, API traffic.

How Slack Handles API Traffic
Internally, a host of specialized internal services work together to manage different aspects of Slack's traffic:
Flannel: An edge cache that holds essential user and channel information.
Imgproxy: Manages image content within Slack.
Supra and Miata: Handle your file uploads and downloads.
After navigating this intricate network, your requests finally land on Slack's WebApp, where you see your messages, files, and integrations.
So, the next time you send a message or share a file on Slack, you'll have a newfound appreciation for the complex but highly efficient system running in the background.
Find the full in-depth analysis here.
WHY DEVELOPERS LOVE RUST: THE RISE OF TECH’S MOST DESIRED LANGUAGE

For an impressive eight consecutive years, Rust has claimed the title of "Most Desired Programming Language" in Stack Overflow's yearly survey of developers.
With over 80% of developers eager to continue using it next year, it's remarkable how this language, founded less than two decades ago, has captivated the global developer community.
In this feature, we'll explore Rust's intriguing history, its typical applications, and the reasons behind its skyrocketing popularity.
The Rust Origin Story
Born out of a need for a safer alternative to C and C++, Rust has rapidly ascended to become a cornerstone in systems programming.
Initially developed by Graydon Hoare in 2006, and later backed by Mozilla in 2009, Rust was a game-changer with its stable 1.0 release in 2015.
Did you know?
The spark for Rust actually came from a malfunctioning elevator experience, inspiring Hoare to create a language that could eliminate common memory issues.
The language prioritizes safety, performance, and productivity, employing static typing to ensure memory safety and reduce errors.
Fast forward to 2023, Rust is the go-to language for about 2.8 million developers, known as "Rustaceans," and has even been embraced by Big Tech companies like Microsoft for its memory-safe capabilities.
The language even has an unofficial mascot, Ferris, adding a whimsical touch to its growing popularity.

Ferris, the Unofficial Mascot for Rust
What Makes Rust Unique
Rust stands out for its unique blend of speed and reliability, addressing developers' memory management challenges often seen in C and C++.
Its ownership system and borrowing model offer built-in support for concurrent programming, eliminating data races and memory issues.
Furthermore, unlike languages that use garbage collection, Rust relies on its ownership rules for efficient memory management, giving developers precise control over resource allocation.
Its package manager, Cargo, not only simplifies project management but also boasts a rich ecosystem of performance-critical libraries.
Rust was the first systems language with a standard package manager, enhancing its robustness.
Other key features include:
Zero-cost abstractions, allowing high-level code without performance loss
Pattern matching for clean data structure handling
Advanced type inference, which automates expression detection based on context.
These combined features make Rust a uniquely powerful and efficient programming language.
Where Rust Excels
Rust's adaptability makes it a top choice for a myriad of applications:
Backend Systems: Its performance and thread safety are invaluable for building performance-critical backend systems, as seen in GitHub's code search feature.
Operating Systems: Rust is a preferred language for OS development, powering systems like Redox OS and Google's Fuchsia.
Web Development: With async programming and high performance, Rust is making waves in server-side applications, supported by frameworks like Rocket.
Emerging Technologies: Rust is carving a niche in cryptocurrency and blockchain technologies, and its minimal runtime makes it ideal for embedded systems and IoT development.
In summary, Rust has captivated developers with its focus on safety, performance, and versatility, earning it a place of honor in the programming world.
Let’s end with something for the Rust aficionados:
fn main() {
break rust;
}
Find the full in-depth analysis here.
HOW LINKEDIN AUTOMATES APPLICATION SECURITY FOR A SAFER USER EXPERIENCE

LinkedIn's Information Security team is committed to creating a secure environment for its users.
At its core is the Application Security team, who manage key security headers like Content Security Policy (CSP) to fortify web applications.
Their mission is to automate security protocols, boost developer productivity, and reduce manual oversight.
They’ve experimented with two architectural models to achieve this:
Centralized, Rule-Heavy Approach: This model scrutinizes outgoing HTTP responses against a set of stringent rules, ensuring they meet the gold standard of security.
Decentralized, Developer-Empowered Model: This approach offers developers the latitude to innovate while maintaining a robust security framework.
Both models deploy rules to LinkedIn's proxy hosts, monitored by specialized plugins. This dual strategy not only fortifies security but also streamlines the development process.
Analyzing the Centralized Approach
Initially, LinkedIn employed a centralized approach, using the Traffic Headers Plugin to write Content Security Policy (CSP) headers into responses.
While this offered benefits like easier maintenance and uniform secure policies, it posed significant challenges as LinkedIn scaled.
The centralized system became increasingly unwieldy, placing the Application Security team in a bottleneck position for approving changes, thereby increasing the risk of human error and site issues.

LinkedIn’s Centralized Architecture Model
Recognizing these limitations, LinkedIn pivoted to a decentralized approach, aligned with the latest CSP standards. This new architecture aimed to achieve five key objectives:
Minimize the impact of changes
Empower developers
Provide a consistent testing experience
Enhance security posture
Build a generalized solution beyond just CSP headers
While the old centralized architecture remains for legacy applications, the focus has shifted towards migrating to a more agile and scalable decentralized system.
Migrating to a New Decentralized System
In this new architecture, a CSP Filter became an integral part of the frontend frameworks.
This filter decorates the outgoing response with CSP headers defined by developers, maintaining a safety net with a fallback mechanism at the traffic layer.

LinkedIn’s Decentralized Architecture Model
This approach reduced the scope and impact of CSP changes, empowering developers to implement and test changes efficiently.
However, the decentralized model posed challenges in security governance, including reduced visibility for the Application Security team and the complexity of CSP modifications.
Implementing Risk-Based Validation Rules
To address these challenges, LinkedIn adopted a "shift-left" approach, implementing security validators that analyze code at the time of commit using risk-based rules.
Validation checks on GitHub guide developers on appropriate actions, such as blocking pull requests that violate key risk rules.

Example of LinkedIn’s Critical Rules
LinkedIn's balanced approach aims to combine the rigor of a centralized system with the flexibility of a decentralized model, making it a safer and more efficient platform for both users and developers.
In summary, LinkedIn's dual approach to application security serves as a compelling case study in balancing security rigor with developmental agility.
Find the full in-depth analysis here.
BYTE-SIZED TOPICS
INTERESTING PRODUCTS, TOOLS & PACKAGES
TIP OF THE WEEK
When it comes to deploying workloads on Kubernetes, defining resource requests and limits for CPU and memory is not just good practice—it's a necessity.
Take a look at this sample pod configuration:
apiVersion: v1
kind: Pod
metadata:
name: my-application
spec:
containers:
- name: my-container
image: my-image
resources:
requests:
memory: "64Mi"
cpu: "250m"
limits:
memory: "128Mi"
cpu: "500m"
Here's four reasons why:
Predictable Resource Allocation: Think of resource requests as RSVPing a table at a restaurant for your pod. It ensures your pod gets the resources it needs to run smoothly.
Avoid Resource Starvation: Setting limits is your node's bouncer, keeping your pod from hogging all the resources and starving others. It's your defense against the "noisy neighbor."
Improve Stability: Without limits, a rogue pod could go on a resource spree, crashing other pods or even the node. It's your speed limit for safe pod driving.
Efficiency: Fine-tuning these values is like optimizing your car for fuel efficiency. You get peak performance without waste.
MEME OF THE WEEK

Reply